class: center, middle, inverse, title-slide # A First Bayesian Course: Why, What, and How ##
bit.ly/dogucu-talks
Talk at RSS Teaching Statistics SIG,
The past, present and future of statistics teaching
### Mine Dogucu, Ph.D. ### 2022-05-04 --- class: middle center <img src="img/headshot.jpeg" alt="A headshot of a woman with curly, short, ear-length hair with green eyes and red lipstick." style="width:165px; margin-top:20px; border: 3px solid whitesmoke; padding: 10px;"> .large[
] <a href = "http://minedogucu.com">minedogucu.com</a> .large[
] <a href = "http://github.com/mdogucu">mdogucu</a> .large[
] <a href = "http://twitter.com/MineDogucu">MineDogucu</a> --- class: center middle <img src="img/stats-115-logo.png" title="Stats 115 course logo with prior, likelihood, and posterior plots!" alt="Stats 115 course logo with prior, likelihood, and posterior plots!" width="25%" style="display: block; margin: auto;" /> [Introduction to Bayesian Data Analysis](https://www.stats115.com) --- <img src="img/bayes-courses-us.png" title="A table with list of majors that include Bayesian courses as part of the major. There are two majors that require a Bayesian course." alt="A table with list of majors that include Bayesian courses as part of the major. There are two majors that require a Bayesian course." width="68%" style="display: block; margin: auto;" /> <a href="https://arxiv.org/abs/2109.00848" style="font-size: 15px">Dogucu, M., & Hu, J. (2021). The Current State of Undergraduate Bayesian Education and Recommendations for the Future. arXiv preprint arXiv:2109.00848.</a> --- class:center <img src="img/bayes-rules-hex.png" title="a hex shaped logo with shiny green-pink disco ball and purple starry background. There is text that says Bayes Rules!" alt="a hex shaped logo with shiny green-pink disco ball and purple starry background. There is text that says Bayes Rules!" width="25%" style="display: block; margin: auto;" /> .pull-left[ <script src="https://use.fontawesome.com/releases/v5.15.1/js/all.js" data-auto-replace-svg="nest"></script> <i class="fas fa-book fa-2x" aria-hidden="true" title="Book icon"></i> [Bayes Rules! An Introduction to Applied Bayesian Modeling](https://bayesrulesbook.com) ] .pull-right[ <i class="fab fa-r-project fa-2x" aria-hidden="true" title="R logo"></i> [{bayesrules}](https://www.github.com/bayes-rules/bayesrules) ] --- class: middle .pull-left[ .center[ <img src="img/alicia.jpg" alt="A headshot of a woman with long blonde hair wearing a brownish yellow tshirt and a red and pink floral silk scarf wrapped around her neck." style="width:165px; margin-top:20px; border: 3px solid whitesmoke; padding: 10px;"> Alicia Johnson .font-20[Macalester College] [
](https://ajohns24.github.io/portfolio) [
](https://github.com/ajohns24) ] ] .pull-right[ .center[ <img src="img/miles.png" alt="A headshot of a man with short dark hair, and a dark moustache. He is wearing a blue button up shirt and dark gray jacket" style="width:165px; margin-top:20px; border: 3px solid whitesmoke; padding: 10px;"> Miles Ott .font-20[Twitter] [
](https://milesott.com/) [
](https://github.com/MilesOtt) [
](https://twitter.com/Miles_Ott) ] ] --- class: middle ## Southern California Data Science Program HDR DSC awards: \#2123366 \#2123380 \#2123384 <img src="img/nsf-logo.png" title="NSF logo" alt="NSF logo" width="10%" style="display: block; margin: auto;" /> Through this collaborative grant between University of California Irvine, California State University Fullerton, and Cypress College, a Bayesian course will be adopted at California State University Fullerton. --- class: middle <style type="text/css"> .panelset { --panel-tab-foreground: whitesmoke; --panel-tab-active-foreground: whitesmoke; --panel-tabs-border-bottom: #00a1a1; --panel-tab-inactive-opacity: 0.5;} </style> .panelset.sideways[ .panel[.panel-name[Unit 1] ### Bayesian Foundations .pull-right[ - Bayes' Rule - The Beta-Binomial Bayesian Model - Balance and Sequentiality in Bayesian Analysis - Conjugate Families ] .pull-left[ <img src="index_files/figure-html/unnamed-chunk-7-1.png" title="three curves on a single plot with no axis labeled. It is coloring scheme indicates its similarity to the previous plot with prior, scaled likelihood and posterior" alt="three curves on a single plot with no axis labeled. It is coloring scheme indicates its similarity to the previous plot with prior, scaled likelihood and posterior" style="display: block; margin: auto;" /> ] ] .panel[.panel-name[Unit 2] ### Posterior Simulation & Analysis .pull-right[ <img src="img/unit2.png" title="A traceplot with no axis labels. Traceplots have thin vertical lines with varying lengths." alt="A traceplot with no axis labels. Traceplots have thin vertical lines with varying lengths." width="100%" style="display: block; margin: auto;" /> ] .pull-left[ - Grid Approximation - The Metropolis-Hastings Algorithm - Posterior Estimation - Posterior Hypothesis Testing - Posterior Prediction ] ] .panel[.panel-name[Unit 3] ### Regression and Classification .pull-right[ <img src="img/unit3.png" title="A scatterplot with multiple regression lines passing through points. These regression lines are not all over the place, they are clustered with similar but varyin intercepts and slopes." alt="A scatterplot with multiple regression lines passing through points. These regression lines are not all over the place, they are clustered with similar but varyin intercepts and slopes." width="100%" style="display: block; margin: auto;" /> ] .pull-left[ - Normal Regression - Poisson and Negative Binomial Regression - Logistic Regression - Naive Bayes Classification ] ] .panel[.panel-name[Unit 4] ### Hierarchical Models .pull-right[ <img src="img/unit4.png" title="a figure showing hierarchy with a rectangle on top. With a set of arrows pointing downwards leading to a set of rectangles below which also have a set of arrows pointing downwards leading to a different set of rectangles." alt="a figure showing hierarchy with a rectangle on top. With a set of arrows pointing downwards leading to a set of rectangles below which also have a set of arrows pointing downwards leading to a different set of rectangles." width="100%" style="display: block; margin: auto;" /> ] .pull-left[ - Normal hierarchical models without predictors - Normal hierarchical models with predictors - Non-Normal Hierarchical Regression & Classification ] ] ] --- class: middle ## Background of students taking the course - Prerequisite: STATS 120C. Introduction to Probability and Statistics III - Recommended: STATS 110. Statistical Methods for Data Analysis I - Students: Data Science major (required), Statistics minor (elective) --- class: middle center ## Big Picture <img src="img/bayes-restaurant.png" width="55%" style="display: block; margin: auto;" /> --- class: middle ## Context In Alison Bechdel’s 1985 comic strip The Rule, a character states that they only see a movie if it satisfies the following three rules ([Bechdel 1986](https://dykestowatchoutfor.com/the-essential-dtwof/)): - the movie has to have at least two women in it; - these two women talk to each other; and - they talk about something besides a man. -- Let `\(\pi\)`, a random value between 0 and 1, denote the unknown proportion of movies that pass the Bechdel test (i.e. `\(\pi \in [0,1]\)`). --- .pull-left[ 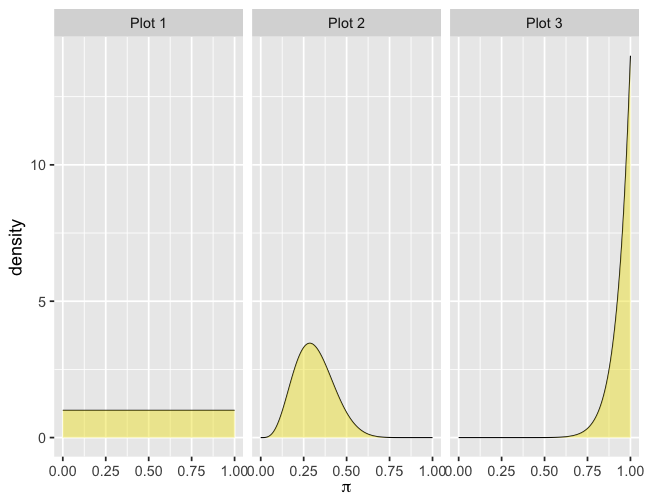<!-- --> ] -- .pull-right2[ - **Feminist** thinks that women are not represented in movies often. - **Clueless** is unsure. - **Optimist** thinks that Bechdel is a low bar for representation of women in movies and thinks almost all movies pass the test. - Can you match the plots with the personas?] --- class: middle ## Plotting Beta Prior with `bayesrules` package One can use the `plot_beta()` function in the `bayesrules` package to try different shape parameters. Example: ```r library(bayesrules) plot_beta(alpha = 2, beta = 10) ``` <img src="index_files/figure-html/unnamed-chunk-14-1.png" style="display: block; margin: auto;" /> --- ## Plotting Beta Prior <img src="index_files/figure-html/unnamed-chunk-15-1.png" style="display: block; margin: auto;" /> --- <img src="index_files/figure-html/unnamed-chunk-17-1.png" style="display: block; margin: auto;" /> --- class: middle ```r one_mh_iteration <- function(w, current){ # STEP 1: Propose the next chain location proposal <- runif(1, min = current - w, max = current + w) # STEP 2: Decide whether or not to go there proposal_plaus <- dnorm(proposal, 0, 1) * dnorm(6.25, proposal, 0.75) current_plaus <- dnorm(current, 0, 1) * dnorm(6.25, current, 0.75) alpha <- min(1, proposal_plaus / current_plaus) next_stop <- sample(c(proposal, current), size = 1, prob = c(alpha, 1-alpha)) # Return the results return(data.frame(proposal, alpha, next_stop)) } ``` --- ```r mh_tour <- function(N, w){ # 1. Start the chain at location 3 current <- 3 # 2. Initialize the simulation mu <- rep(0, N) # 3. Simulate N Markov chain stops for(i in 1:N){ # Simulate one iteration sim <- one_mh_iteration(w = w, current = current) # Record next location mu[i] <- sim$next_stop # Reset the current location current <- sim$next_stop } # 4. Return the chain locations return(data.frame(iteration = c(1:N), mu)) } ``` --- ```r set.seed(84735) mh_simulation_1 <- mh_tour(N = 5000, w = 1) ``` .pull-left[ 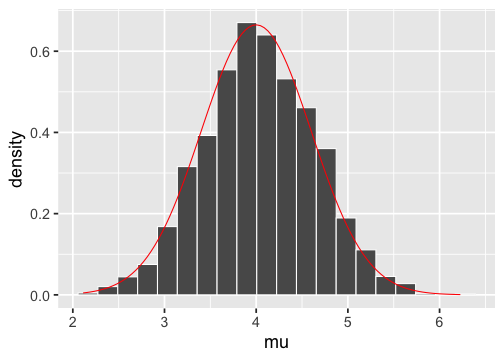<!-- --> ] .pull-right[ 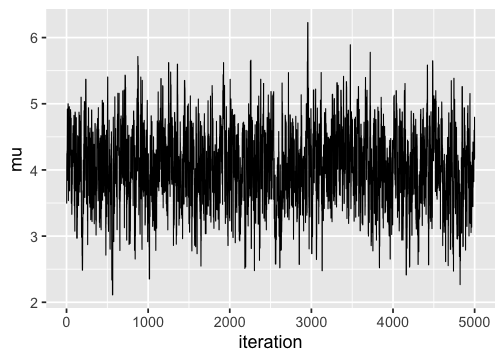<!-- --> ] --- class: middle ## `library(rstan)` .pull-left[ ```r # STEP 1: DEFINE the model stan_bike_model <- " data { int<lower=0> n; vector[n] Y; vector[n] X; } parameters { real beta0; real beta1; real<lower=0> sigma; } model { Y ~ normal(beta0 + beta1 * X, sigma); } " ``` ] .pull-right[ ```r # STEP 2: SIMULATE the posterior stan_bike_sim <- stan(model_code = stan_bike_model, data = list(n = nrow(bikes), Y = bikes$rides, X = bikes$temp_feel), chains = 4, iter = 5000*2, seed = 84735) ``` ] --- class: middle ## `library(rstanarm)` ```r normal_model_sim <- stan_glm(rides ~ temp_feel, data = bikes, family = gaussian, chains = 4, iter = 5000*2, seed = 84735) ``` --- class: middle ## Pedagogical Approach - Checking intuition -- - Active learning (quizzes and applications) -- - Computing & math together -- - Compute for a single case, then use built-in functions -- - [Accessible and Inclusive](https://arxiv.org/abs/2110.06355) --- class: middle - [Undergraduate Bayesian Education Resources](https://undergrad-bayes.netlify.app/) -- - [Undergraduate Bayesian Education Network](https://undergrad-bayes.netlify.app/network.html) --- class: middle center # THANK YOU .large[ [bit.ly/dogucu-talks](https://bit.ly/dogucu-talks) ] .large[
] <a href = "http://twitter.com/MineDogucu">MineDogucu</a>